Multi-Robot (Swarm) Coordination
Teaming of Bounded Rational Agents
Our lab has also been working on the intricate dynamics of multi-agent systems, where robots must not only operate in concert with their robotic counterparts but also collaborate effectively with human partners. In environments where multiple intelligent agents coexist—be they other robots or human operators—comprehending the behaviors of neighboring agents becomes essential for achieving efficient task completion. The chal- lenge, however, is that these agents typically do not transparently communicate their decisions or reasoning processes, necessitating that robots autonomously deduce the implications of others’ actions. This level of reasoning can place significant demands on computational resources. In many practical applications, achieving the absolute optimal behavior from every agent is not always feasible or necessary. Instead, it is often more advantageous to develop solutions that offer sub-optimal but sufficiently effective performance while remaining computationally manageable. This synthesis is made possible by forging connections between economic-theoretic and decision-theoretic frameworks. We have developed such coordination mechansim based on bounded rationality analysis.
YouTube link:
Heterogeneous Multi-Robot Systems
We are also interested in hybrid systems (both hardware and software). For example, an Unmanned Aerial Vehicle (UAV) carrying a miniature Unmanned Ground Vehicle (miniUGV) to perform a complex search and manipulation task. Such system can leverage heterogeneous robots to accomplish a task that cannot be done using a single robot system. It enables the UAV to explore a hidden space with a narrow opening through which the miniUGV can easily enter and escape. The hidden space is assumed to be navigable for the miniUGV. The miniUGV uses Infrared (IR) sensors and a monocular camera search for an object in the hidden space. The proposed system takes advantage of a wider field of view (fov) of the camera as well as the stochastic nature of the object detection algorithms to guide the miniUGV in the hidden space to find the object. Upon finding the object the miniUGV grabs it using visual servoing and then returns back to its start point from where the UAV retracts it back and transports the object to a safe place. In case there is no object found in the hidden space, UAV continues the aerial search. The tethered miniUGV gives the UAV an ability to act beyond its reach and perform a search and manipulation task which was not possible before for any of the robots individually. The system has a wide range of applications and we have demonstrated its feasibility through repetitive experiments.YouTube link:
Swarm Formation Control
An important ramification of swarm control research involves manoeuvring a spatially dispersed system from one formation to another. We consider the problem of changing smoothly between formations of spatially deployed swarm systems. We are interested in addressing scenarios in which gradual and seamless formation transitions are needed, a problem which we term "formation morphing". We show that this can be achieved by routing agents on a Euclidean graph that corresponds to paths computed on - and projected from - an underlying three-dimensional matching graph. The three-dimensional matching graph is advantageous in that it simultaneously represents a logical assignment problem (for which an optimal solution must be sought) and metric information that comprises the spatial aspects of the Euclidean graph.
Figures above: a swarm of drones moving across some narrow space.
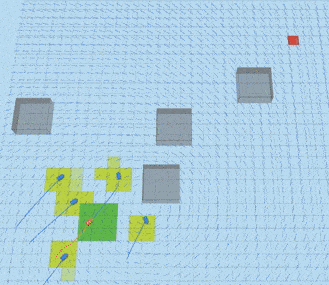
It is challenging for an autonomous robot to make decisions in a dynamic environment in the presence of other moving vehicles. Decisions of the robot must be computed fast to cope with uncertain or disrupting behaviors of other vehicles (either autonomous or non-autonomous, but are not under our control). To overcome this challenge, the robot planning requires certain look-ahead knowledge of the dynamics for both our robot and other vehicles. We first predict the future state distributions of other vehicles to account for their uncertain behaviors affected by the time-varying disturbances. We then construct a dynamic-obstacle-aware reachable space that contains states with high probabilities to be reached by the robot, within which the optimal action policy is searched. Since, in general, the dynamics of both the vehicle and the environmental disturbances are nonlinear, we utilize a nonlinear Gaussian filter – the unscented transform – to approximate the future state distributions.
Figures above: five micro aerial vehicles fly in certain formations (GIF speed x2).
We are also interested in constrained coordination problems, where the constraints can be from the multi-robot systems and/or from the external environments. For instance, we worked on energy-constrained planning problems, where a team of aerial vehicles is deployed to pursue an objective such as surveillance, monitoring, or exploration of an indoor environment with cognizance of available onboard energy resources and pre-deployed recharging platforms. The method can be extended to other applications, for example, drone pacakge deliveries. Our work focuses on deployments which seek to balance individual and cooperative vehicle task requirements, with overall travel and energy costs and charging station availability, toward enabling extended duration operation.
Decentralized Multi-Robot Coordination via Task Allocation
We are interested in scalable approaches for multi-robot coordination. One fundamental framework to achieve this goal is the decentralized or distributed task allocation. There are several open challenges that complicate designing—especially analyzing—decentralized algorithms. For example, the information attained by individual robots may be incomplete and inaccurate, resources can be limited, communication can be constrained, and action decisions may have to be conservative due to sub-optimal or uncertain results. Within the distributed context, I have been particularly interested in improving performance in terms of computation and communication complexities, solution quality, robustness, hierarchical flexibility, etc.
We have developed an extremely fast sensitivity analysis method for classic assignment problem (O(n^2) time for each variable). It is achieved through modifying the matching graph operations of the well-known Hungarian algorithm, so that the standard computation is relaxed and bounds are produced. Different from existing uncertainty analysis approaches most of which utilize the stochastic means, this algorithm is a deterministic method with bounded time complexity.
iRobots with particle-filter SLAM used to analyze sensitivity of task allocation.
The robots we used are the create type made by iRobot, as shown right figure. A Hokuyo laser range sensor is mounted on each robot which records range data up to ∼5 m and an ASUS netbook is carried by each robot to compute all data including the communication, utility estimation, interval analysis, logging, etc.